Biased Algorithms, Biased World
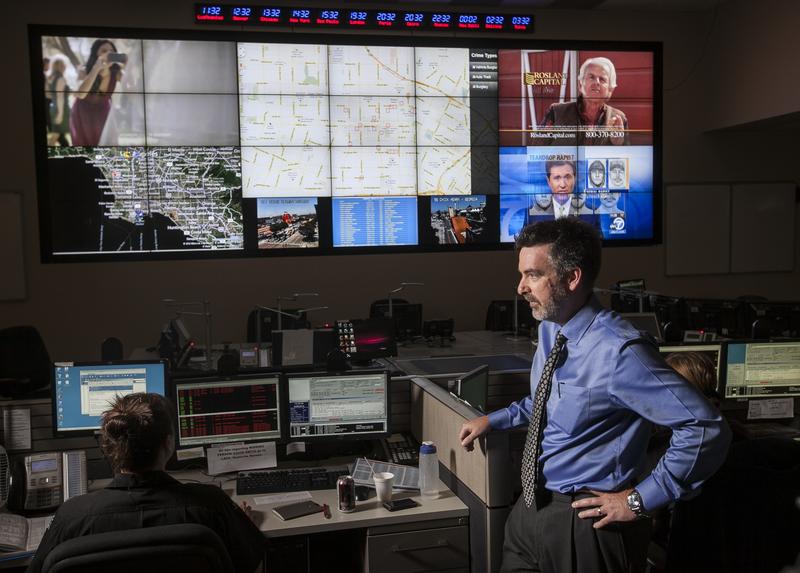
( Damian Dovarganes / AP Images )
Brooke Gladstone: This is On the Media. I'm Brooke Gladstone. While Congress sweats it out during their summer recess, we decided to take a break from politics, take refuge in the uncontroversial world of numbers. After all, addition is neutral, right? For much of the 21st century, it seemed that if you had a problem, you could just throw an algorithm at it. Algorithms are replacing human advisors and brokers.
Speaker 1: Our military uses an algorithm in their Skynet program to decide who should be on the terrorism kill list.
Speaker 2: AI is being used for everything, from diagnosing illnesses, to helping police predict crime hotspots.
Brooke: The only problem, a lot of times they just don't work. Stanley Tucci may have said it best in the midst of a robotic production snafu in the movie Transformers: Age of Extinction.
Stanley Tucci: Algorithms, Math, why can't we make what we want to make the way we want to make it? Why?
Brooke: Yes, why? Well, in 2019, we sat down with slightly less dramatic flair to ask Cathy O'Neil, mathematician, data scientists, and investigative journalists that very question. She founded the consulting firm, ORCAA, which audits algorithms for racial, gender, and economic inequality and all-around bad science. She loves math. She used to be a wall street quant.
Something about the financial meltdown of 2008, turned her off the use of algorithms for the purposes of prediction, something about how no one actually checks to see if they really work and what happens when they don't and even when they do. She's the author of Weapons of Math Destruction: How Big Data Increases Inequality and Threatens Democracy. I started with the basics. What's an algorithm?
Cathy O'Neil: It's just a set of directions, a long division that you learn in fourth grade is an algorithm. I use the word algorithm, it's short for predictive algorithm and that's a way of predicting the future based on the past. We use the training data that is all around us.
Brooke: When you say training data--
Cathy: The information we've collected from the past, like its memories.
Brooke: They are used to select to whom to give or deny alone?
Cathy: That's right.
Brooke: Who gets hired and who doesn't?
Cathy: Who goes to prison and for how long.
Brooke: There are algorithms and there are weapons of math destruction. What's the difference?
Cathy: Most algorithms are totally benign. I could build an algorithm in my basement on my computer. I could be trying to predict the stock market. Nobody uses it, it doesn't matter. I guess the shorthand version is algorithms that are important, that are destructive, and that are secret. That's the weapon of math destruction.
Brooke: Let's talk about some algorithms that you note in your book that are maybe one of those things, but not the others. For instance, sports algorithms, predicting how teams or players may behave, fed data that actually reflect the behavior that they're trying to predict. They are regularly updated. Though they are widely used, you wouldn't regard them as a weapon of math destruction.
Cathy: Correct. They're very important in the sense that there's a lot of money behind them and people really care if they're right or wrong. If they make a mistake, that gets learned. If we don't trade for a player, and they go to another team and do really well, the algorithm learns that they made a mistake. That's often not the case for weapons of math destruction. The real thing that distinguishes that is that it doesn't wreak havoc.
Brooke: Can you give me an example of using proxies? You can't actually use the real thing like an athlete's behavior in the league, you have to use something that might be an indicator of something else. That's a proxy that characterizes a lot of your WMD.
Cathy: Absolutely.
Brooke: Give me an example of that.
Cathy: Well, the most pernicious example of that is, in my opinion, the predictive policing or the crime rate score, the recidivism risk scores.
Speaker 3: Police in Los Angeles are trying to predict the future.
Speaker 4: We know where crime happened yesterday, but where is it going to happen tomorrow in the next day?
Speaker 3: They're not alone. More and more departments are using data-driven algorithms to forecast crime.
Cathy: They predict locations of arrests, to say that's where the crime must be, rather than acknowledging that police act differently in certain neighborhoods than they act in other neighborhoods. We don't really have crime data. If you think about it, there's lots of crimes that go on that do not lead to arrests. There's lots of pot smoking among white people, but never get arrested. There's a lot more non arrested white crime than people of color. When we use arrest as a proxy for crime, we are really overburdening those people who are already profiled by the police.
Brooke: In that case, arrests are used instead of criminal behavior?
Cathy: Yes. When I say something like arrests are a bad proxy for crime, I'm sure a lot of your listeners are like, "But people get arrested for crimes." I just want to make a point that, I've talked to a lot of police chiefs and a lot of judges about these kinds of algorithms and one of the things they keep on coming back to is almost no real mental health care in this country. People get arrested very consistently for addiction problems or untreated mental health problems. That's not a crime.
The police don't think it's crime, the judges don't really want to think of it as a crime and yet, these scoring systems are basically suggesting, since this person is much more likely to be rearrested in the future because guess what, they're still going to be addicted or they're still going to have a mental health problem or they're still going to be poor, and there's all sorts of crime
[crosstalk]
Brooke: They're still going to be living in the neighborhood where behavior that could go on pretty much unchallenged and the frat house is going to send you into the system?
Cathy: Exactly. Those very predictable things show up quite well, statistically. That is how your score goes up. That's how you get to that point where you're considered high risk, and you're actually sentenced to prison for longer. The judges don't like it. The judges want to be putting people into prison because they're actually a public health risk, not because they're addicts.
Brooke: That's a proxy, that characterizes a lot of your WMD.
Cathy: Everybody will understand hiring algorithms. Let's just say you have a big company, Brooke. You're just like, "Oh, my God, I'm getting so many applications for these 10 positions and I get 1,000 applications. How am I going to sort through them all? I would like somebody to help me. I don't want it to be in person because they're too expensive. I want it to be an algorithm."
You hire me. I'm your data scientist and I come and build you an algorithm to sort through these applications. Where you're going to say, "Cathy, I want to hire people that will be successful at my firm." I'll say, "Okay, well, what do you mean by success?" This is where the proxy comes in. You'll say, "Well, I don't measure directly, whether someone's good at their job."
Brooke: Because you can't know that.
Cathy: Because how do you know that? What does it mean for someone to do well, at your company? What do you think?
Brooke: Okay, they stay.
Cathy: They stay a long time.
Brooke: They generate a lot of good ideas.
Cathy: How do you measure that, though?
Brooke: Let's see. They get promoted?
Cathy: Excellent. Okay, so we have this triumvirate of data points about each employee, like how long do they stay, how many promotions, how many raises. This is exactly the way that people measure success at companies and this is exactly the kind of algorithm that gets built. I'm training your data on 20 years of your past practice and hiring people. Boom, implicit bias that we know exists in who gets promoted, who gets hired, who gets raises, who stays for a long time, who feels welcome enough to stay for a long time, gets baked into the algorithm that I just wrote.
Brooke: You observe in the book that almost all of these algorithms predict behavior, not on what you do or what you've done, because that's so hard to measure but who you are.
Cathy: I'll just add one last point to emphasize how invisible this might look from the perspective of the employer. Certain mistakes are much more obvious when you do it this way, namely, false positives, which is to say, "I've hired someone. They didn't work out." That is easy to spot, because what a pain? What you don't see are the people that you could have hired that would have worked out, but were filtered out. That's where we see the narrowing bottleneck of who is deemed future successful.
Brooke: Another thing we don't really understand how it works, but we have to worry about its implications is facial recognition technology. What's the problem there?
Cathy: Well, there's a bunch of different levels of problems. One of them is that it sometimes just doesn't work. My friend, Joy Buolamwini, at MIT Media Lab was the first to come out with an audit. She's done a few audits now at Amazon, most recently, where she found that at a technical level, they weren't working very well.
Joy Buolamwini: All companies performed better on males than females and all companies also performed better on lighter subjects than on darker subjects. We saw that all companies performed the worst on darker females. In fact, as we tested women with darker and darker skin, the chances of being correctly gendered can close to a coin toss.
Brooke: Why? Why does facial recognition work better on white men and Black women?
Cathy: It doesn't have to. It just happens to because of the training data. Literally, the corpus of pictures that were used to train the algorithm was much more white and much more male. I think Joy calls up the pale male data problem. Believe it or not, they weren't thinking carefully enough before deploying it to the world to say, "Hey, does this work as well on black faces as white faces?" Why don't these companies get ahead of this a little bit and test this and have evidence in advance that this is not going to be unfair.
Brooke: Who determines if the algorithms are working, and how?
Cathy: That's the craziest part. I'm so glad you asked. Nobody. There is no standard. A large company says, "Oh, we don't want to build these algorithms. We want to rent them. Essentially, we licensed them from some data vendor." The data vendor says, "Oh, you can trust this, but we're not going to explain it at all. It's a Black box."
Brooke: Proprietary.
Cathy: That's part of the licensing agreement. You don't get to know how this works, but you can use it to hire people. You can use it if you're a police department to find people, you can use it if you're a department of education to fire your teachers, blah, blah, blah. There is no particular standard.
Brooke: Which brings us to the issue of, how do you determine when an algorithm is successful? What is your definition of success? I was really moved by your discussion of clopenings.
Cathy: This is a great example of where the definition of success for the people using the algorithm is the opposite as a definition of success for the people who are targeted by the algorithm. Clopenings is the concept where you are basically a minimum wage worker, probably working in a large store, and you close on one evening and then you open the store the next morning. You probably don't have enough time to even go home and see your kids. Barely enough time to sleep.
The crazy thing is that these scheduling algorithms will for one week make you clopen for this in a row, and then the next week you don't have any work at all. I looked into the research that was developing these algorithms. One of them made me cry. It was so brutal. It was like, you have the option, if you use this algorithm to toggle this switch, to make sure that none of your employees get enough hours a week to qualify for benefits.
You can just turn this little switch on, and all of your employees will be wage slaves forever. They will not be able to go to night school because their hours change every day. They will not be able to put their kids in daycare regularly. It is such a small benefit for the employer. If you compare it to the wrecking of the life of the employee, it's maddening, but it's not actually technically illegal. The algorithm exploits it.
Brooke: You pose the question, should we as a society be willing to sacrifice a little efficiency in the interest of fairness. You talk about Starbucks. Starbucks wants to have a good image. Its scheduling algorithm was exposed. It said that it was going to improve it. No more clopenings, no more employing people short of triggering some benefits, but the trouble was that the incentives to managers to be efficient were so irresistible that they never actually made any changes.
Cathy: Yes, it's philosophical question. Basically, you're saying, do we have any answer to capitalism? All these algorithms that they're using in these corporate settings are about profitability, not about happiness. If we wanted to address that, we would actually have to change the incentive structure of corporations. It's a big task.
Brooke: How would you assess the way that we, the general public, view algorithms?
Cathy: I want us to learn to be skeptical. I want us to say, "I don't need a math PhD to ask you why I'm getting fired." The power that we give to the algorithms is the thing we have the most control over.
Brooke: Do we?
Cathy: Let me give you another example. The US News & World Report College Ranking model, who gives that power? Us.
Brooke: You describe the impact of that ranking. Colleges turn themselves into pretzels. Students spend tons of money in order to fit the parameters that colleges have adapted to because of the rankings in US News & World Report, a pernicious feedback loop, you call it.
Cathy: The bogus and they're gainable. The college knows that if they look exclusive, then they look better for the ranking. They just get a bunch of kids. They know we'll never make it to apply. Yes, of all the stupid things that the US News & World Report pays attention to, it doesn't pay attention to the costs.
Brooke: It's not one of the criteria exactly?
Cathy: Exactly. When college admissions officers are crazily gaming the algorithm, which is what their job seems to be nowadays, they don't care if the tuition goes up, why do we keep giving these questionable, stupid algorithms so much power?
Brooke: If people listening to this interview only take one thing away, this is what I'd want them to take away. You say that an algorithm is an opinion embedded in math?
Cathy: Right. There's so many choices that go into every algorithm, and the most important one being, what do we mean by success? If I get to define success for myself, that's one thing. If Facebook is defining success for me, I don't trust it as algorithms proliferate, which they are, applying for credit, applying for a job, applying to go to college, applying for a loan, applying for housing, all those things are now algorithmic. They all define success for them, not for us. That's their opinion. It's really, really important to remember that it's not necessarily opinions that you have to share.
Brooke: Thank you so much.
Cathy: Thank you so much, Brooke.
[music]
Brooke: We spoke with Cathy O'Neil in 2019, she is a mathematician, data scientist, founder of the consulting firm, ORCAA, and the author of Weapons of Math Destruction: How Big Data Increase An Inequality and Threaten Democracy.
[music]
Copyright © 2021 New York Public Radio. All rights reserved. Visit our website terms of use at www.wnyc.org for further information.
New York Public Radio transcripts are created on a rush deadline, often by contractors. This text may not be in its final form and may be updated or revised in the future. Accuracy and availability may vary. The authoritative record of New York Public Radio’s programming is the audio record.